The realm of data science is in a constant state of evolution and transformation, as new technologies emerge to streamline the process of working with data. One such innovation is no-code AI, which promises to allow users to build complex machine learning models without any programming background. While no-code AI may be convenient for non-programmers, it is crucial to acknowledge that this tool also has numerous limitations that cannot be ignored. In this comprehensive blog post, we will delve into the most pressing limitations of no-code AI and challenge the notion that it is a silver bullet for all data science problems.
- Lack of Flexibility: Perhaps the most significant drawback of no-code AI is its limited flexibility in comparison to traditional coding. This restriction can cause significant roadblocks for data scientists who need to adapt their models quickly to changes in business requirements or new data.
- Limited Functionality: No-code AI offers only a fraction of the functionality available in traditional coding languages, making it challenging sometimes to develop complex models that accurately capture all the nuances of data. This limitation could have an impact depending on the use case.
- Debugging Challenges: Debugging is an essential aspect of the data science process, and No-code AI makes it significantly more challenging to debug models compared to traditional coding methods. The complex interplay between the various components of no-code AI models often makes it difficult to identify the root cause of problems.
- The Black Box Paradox: The most significant criticism of no-code AI is that it often results in "black box" models, which are challenging to understand and explain. This lack of transparency raises numerous concerns, both from a business and ethical standpoint. It is challenging to explain to stakeholders why a particular decision was made, and there is no way to guarantee that the model is not biased. This is changing quickly with more investment in explainable AI tooling.
No-code AI presents several advantages, including enabling non-programmers to develop machine learning models. However, these advantages come at a price, as No-code AI also has several limitations to consider. Data scientists must consider the pros and cons of no-code AI thoroughly before deciding whether or not to use it in their projects. The limitations of no-code AI raise questions about its effectiveness and sustainability, and it is imperative that the data science community continues to challenge and push the boundaries of this technology to achieve even better results.
Despite its limitations, No-code AI tools still offer significant benefits
The elimination of the need for programming knowledge means that organizations can train and deploy models more quickly and efficiently, which can lead to faster ROI. Secondly, no-code AI platforms often come with pre-built templates and workflows, making it easier for users to get started and reducing the time it takes to develop models. Bringing AI to the masses, no-code tools are best used for traditional AI use cases.
Moreover, no-code AI tools can also provide valuable insights and predictions in real-time, which can be incredibly beneficial for businesses that need to make quick decisions based on the latest data. Additionally, no-code AI tools are designed to be user-friendly, which can make it easier for businesses to scale AI initiatives and involve more people in the process.
No-code AI tools have the potential to provide significant ROI for businesses.
In my opinion, despite the limitations, No-code AI is a game-changer for businesses of all sizes.
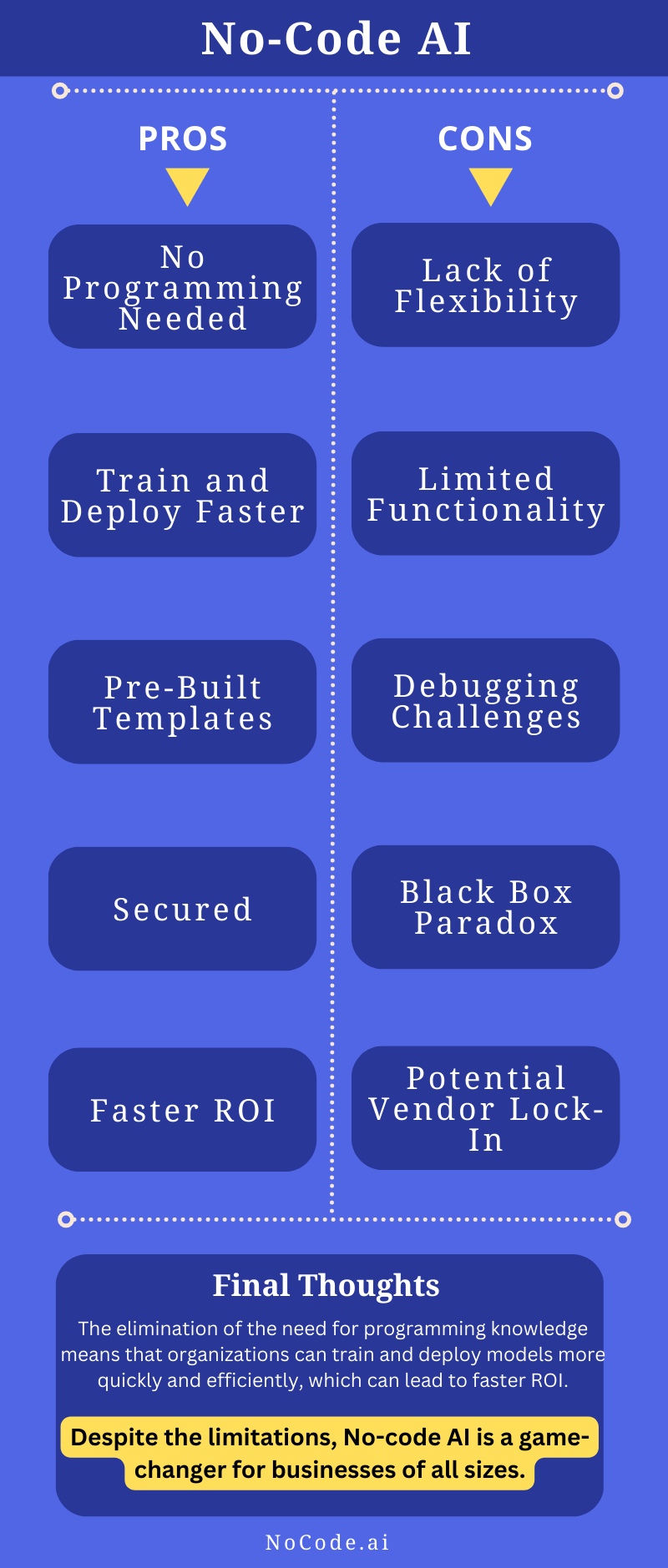